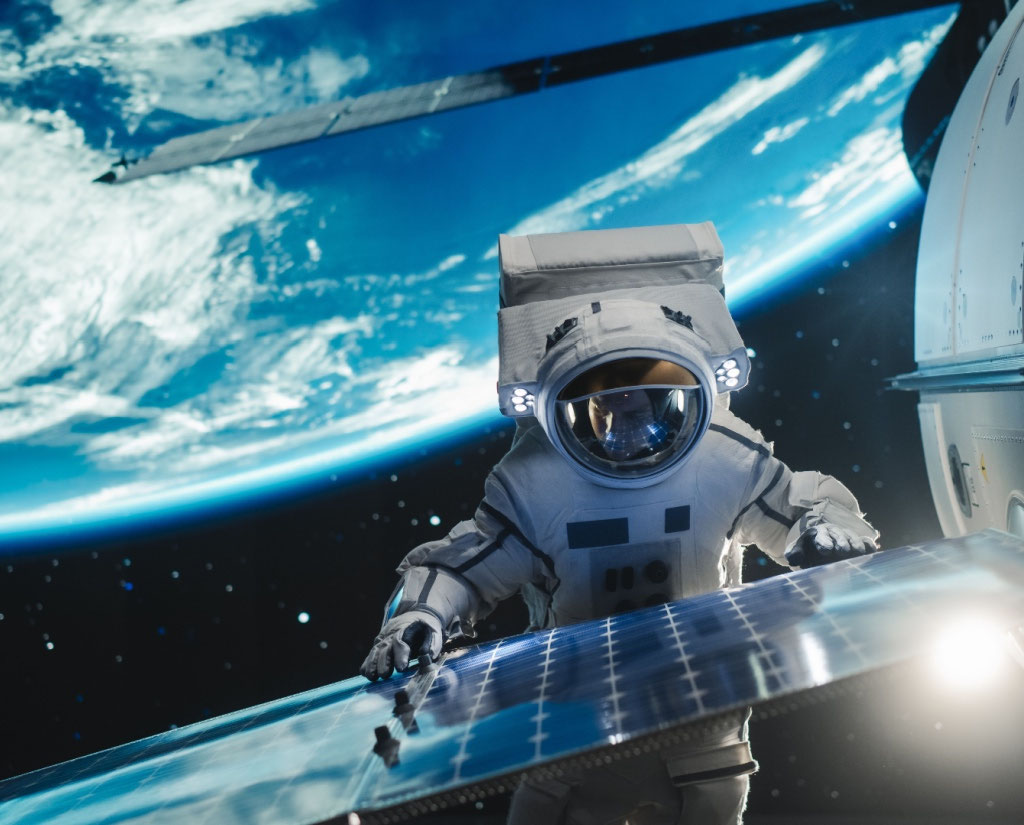
New and emerging technologies have led to the accumulation and exponential growth of vast amounts of information, often referred to as ‘big data’. The ability to create huge data sets is revolutionizing every facet of society, including public health generally and nutrition outcomes specifically. The problem, of course, is what to do with all that information. Luckily, the field of data analytics is advancing almost as fast as the ability to collect information. Data analytics is being used to improve global nutrition outcomes by identifying trends and patterns across populations, focusing attention on specific problem areas, and measuring the effectiveness of food and nutrition policies and programs. Innovative data-driven approaches are enhancing food accessibility and nutritional outcomes for groups and individuals alike.
How Data Analytics Is Used To Improve Nutrition Outcomes
Data analytics can be defined as the use of statistical and computational techniques to organize, study, and interpret large data sets in order to identify emerging trends, patterns, and relationships within the data. The results can then be used to draw actionable insights and conclusions to improve systems and processes and to aid in decision-making. Data-driven approaches and initiatives are essential to improving public health and nutrition outcomes and can be used for a wide variety of specific purposes, including:
- Nutrient analysis of both traditional and emerging food resources to aid public health researchers in creating and enhancing dietary recommendations and guidelines and designing more effective food labeling systems.
- Analysis of dietary habits, trends, and patterns across populations to identify areas in need of improvement and to target critical nutritional interventions.
- Analysis of individual health data to create personalized diet and nutrition plans and to help individuals make better decisions about their food choices.
An example of an emerging field of research that utilizes all three of these approaches is precision nutrition, also called personalized nutrition. It seeks to prevent or treat disease on an individual level using personalized nutritional plans based on characteristics such as age, lifestyle, race, gender, ethnicity, health history, and DNA. It is derived from the recognition that each individual’s body responds to specific foods and nutrients in different ways, making a one-size-fits-all approach to nutrition less effective. Data analysis of nutritional effects on unique characteristics can allow for more precision in dietary treatments for disease.
Challenges And Obstacles To Nutritional Data Analysis
The collection and accurate analysis of information has to contend with several potential problems, especially when it comes to public health research.
Poor data quality - Data that is biased, inconsistent, or incomplete will generate flawed analyses and inaccurate insights, leading to erroneous conclusions and actions. The solution is to gather and store information in a consistent and standardized way and to regularly audit data collection and analysis methodology and integrity.
Insufficient resources - Like other research initiatives, data analytics can require significant amounts of funding and personnel. The pressure can be relieved by making better use of existing resources and cultivating mutually beneficial collaborative partnerships.
Privacy concerns - Data analytics in pursuit of improved health and nutrition outcomes often involves working with personal health data and guaranteeing the protection of that data is essential for maintaining trust with populations and individuals. Data should be anonymized and stored securely in accordance with the relevant privacy laws and regulations.
Data Analytics Initiatives That Have Improved Nutrition Outcomes
There are many examples of research initiatives that have successfully utilized data analytics to improve nutrition outcomes. Here are a few from around the world.
- The USDA’s Economic Research Service oversaw the National Household Food Acquisition and Purchase Survey (FoodAPS), the first nationwide comprehensive survey of U.S. household food purchases. The analysis of the data collected produced insights into food spending trends, food security, and quality of diet. These insights were used to improve nutrition policies and programs and to study relationships between health and diet.
- The POSHAN Abhiyaan initiative in India involves extensive use of data analytics to identify districts with high rates of malnutrition to better target nutrition interventions and has been very successful in improving food security and health outcomes in the poorest communities.
- The Brazilian School Feeding Program is Brazil's oldest public policy initiative, begun in 1954, and the second largest program of its kind in the world. It requires that 30% of the food used in school meals be sourced from local farms and that school menus adhere to official dietary standards. Data analysis is used to monitor the nutritional content of meals and measure health results. It has been successful both in improving nutrition outcomes and promoting rural farming development.
If you have an innovative idea on how to use data analytics to improve nutrition outcomes, register for the ARSX2023 Challenge and submit your solution today.
References:
- National Health and Nutrition Examination Survey (NHANES): https://www.cdc.gov/nchs/nhanes/index.htm
- USDA Nutrient Data Laboratory: https://www.ars.usda.gov/northeast-area/beltsville-md-bhnrc/beltsville-human-nutrition-research-center/nutrient-data-laboratory/
- Challenges in implementing data analytics in healthcare: https://www.ncbi.nlm.nih.gov/pmc/articles/PMC6107669/
- Overcoming challenges in data analytics: https://www.techopedia.com/3-challenges-of-data-analytics-and-how-to-overcome-them/2/33049
- FoodAPS National Household Food Acquisition and Purchase Survey: https://www.ers.usda.gov/data-products/foodaps-national-household-food-acquisition-and-purchase-survey/
- Indian POSHAN Abhiyaan: https://www.india.gov.in/spotlight/poshan-abhiyaan-pms-overarching-scheme-holistic-nourishment
- The Brazilian School Feeding Program: https://www.cambridge.org/core/journals/public-health-nutrition/article/brazilian-school-feeding-programme-an-example-of-an-integrated-programme-in-support-of-food-and-nutrition-security/4245C868F05FC9E7FA43F9CACEF24A1B